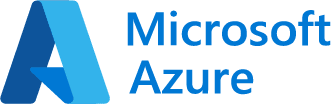
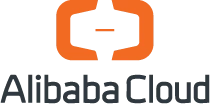
Trusted by
ML/AI Leaderboards
Observability and control
Automation
ML/AI cost optimization
Enhance the ML process in your company with OptScale capabilities, including:
ML/AI Leaderboards provide versioning of model training experiments and rank ML tasks based on metrics. OptScale’s Evaluation Protocol, with a set of rules by which candidates are compared, ensures that trained models are tested consistently and enforces an apples-to-apples comparison.
With OptScale, ML specialists gain full transparency across ML tasks, models, artifacts, and datasets. The OptScale dashboard provides a comprehensive view of various training metrics for each ML model in a single pane of glass, offering model tracking and visualization, as well as cost and performance tracking.
OptScale’s integration with Airflow, Jenkins, and GitHub Actions in MLOps is designed to automate the entire machine learning lifecycle.
By leveraging automation, users can maintain consistency and efficiency across their ML/AI projects. OptScale’s user-friendly interface enables users to monitor effortlessly and schedule model training jobs, manage dependencies and orchestrate ML workflows.
By integrating with the ML/AI model training process, OptScale highlights bottlenecks and offers clear recommendations to reach optimal performance and infrastructure cost, including:
We run a FinOps community with 11,000+ members
Supported platforms
A full description of OptScale as an MLOps open source platform.
Enhance the ML process in your company with OptScale capabilities, including
Find out how to:
Powered by