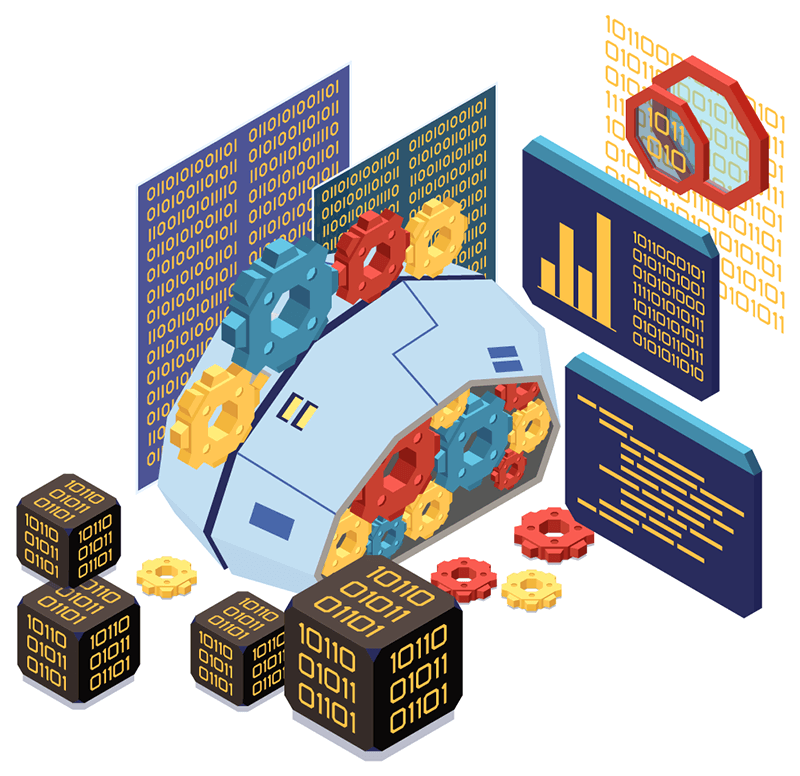
What constitutes hyperparameter tuning?
Hyperparameter tuning refers to the meticulous process of selecting the most effective set of hyperparameters for a given machine-learning model. This phase holds considerable significance within the model development trajectory, given that hyperparameter choice can profoundly influence the model’s performance.
Various methodologies exist for optimizing machine learning models, distinguishing between model-centric and data-centric approaches. Model-centric approaches concentrate on the inherent characteristics of the model itself, encompassing factors like model structure and algorithmic choices. Typically, these methods entail exploring optimal hyperparameter combinations from a predefined set of potential values.
Exploring hyperparameter space and distributions
The hyperparameter space encompasses all potential hyperparameter combinations applicable to training a machine learning model, constituting a multi-dimensional arena where each dimension corresponds to a distinct hyperparameter. To illustrate, hyperparameters such as the learning rate would give rise to a two-dimensional hyperparameter space – one dimension for the learning rate and another for the number of hidden layers.
The distribution delineates the range of values for each hyperparameter and the associated probabilities within the hyperparameter space. It characterizes how likely each value is to occur within space.
- Objective of hyperparameter tuning: The primary goal is to enhance the model’s overall performance. Achieving this involves meticulously exploring the hyperparameter space to pinpoint the combination that brings out the best in the model.
- Impact of hyperparameter distribution: The search process’s effectiveness is shaped by hyperparameter distribution. This decision not only determines the range of values under scrutiny but also assigns probabilities to each value, influencing the tuning strategy and, consequently, the final model performance.
Types of hyperparameter distributions in machine learning
Diverse probability distributions are crucial in defining the hyperparameter space in machine learning. These distributions establish the potential range of values for each hyperparameter and govern the likelihood of specific values occurring.
Log-normal distribution
- Characterized by a logarithmically normal distribution of a random variable.
- Preferred for positive variables with skewed values, enabling a broader range of possibilities.
Gaussian distribution
Symmetrical around its mean, this continuous distribution is commonly used for variables influenced by numerous factors.
Uniform distribution
- Equally likely to select any value within a specified range.
- Applied when the range of potential values is known, and there is no preference for one value over another.
Beyond these, various other probability distributions are found to be applicable in machine learning, such as the exponential, gamma, and beta distributions. The careful selection of a probability distribution significantly impacts the effectiveness of the hyperparameter search, influencing the explored value range and the likelihood of selecting each specific value.
Hyperparameter optimization methods
1. Grid search overview
Grid search is a hyperparameter tuning technique where the model is trained for every conceivable combination of hyperparameters within a predefined set.
To implement grid search, the data scientist or machine learning engineer specifies a set of potential values for each hyperparameter. The algorithm then systematically explores all possible combinations of these values. For instance, if hyperparameters involve the learning rate and the number of hidden layers in a neural network, grid search would systematically try all combinations – like a learning rate of 0.1 with one hidden layer, 0.1 with two hidden layers, etc.
The model undergoes training and evaluation for each hyperparameter combination using a predetermined metric, such as accuracy or F1 score. The combination yielding the best model performance is selected as the optimal set of hyperparameters.
2. Bayesian optimization overview
Bayesian optimization is a hyperparameter tuning approach that leverages Bayesian optimization techniques to discover a machine learning model’s optimal combination of hyperparameters.
Bayesian optimization operates by constructing a probabilistic model of the objective function, which, in this context, represents the machine learning model’s performance. This model is built based on the hyperparameter values tested thus far. The predictive model is then utilized to suggest the next set of hyperparameters to try, emphasizing expected improvements in model performance. This iterative process continues until the optimal set of hyperparameters is identified.
Key advantage:
One notable advantage of Bayesian optimization is its ability to leverage any available information about the objective function. This includes prior evaluations of model performance and constraints on hyperparameter values. This adaptability enables more efficient exploration of the hyperparameter space, facilitating the discovery of the optimal hyperparameter combination.
3. Manual search overview
Manual search is a hyperparameter tuning approach in which the data scientist or machine learning engineer manually selects and adjusts the model’s hyperparameters. Typically employed in scenarios with limited hyperparameters and a straightforward model, this method offers meticulous control over the tuning process.
In implementing the manual search method, the data scientist outlines a set of potential values for each hyperparameter. Subsequently, these values are manually selected and adjusted until satisfactory model performance is achieved. For instance, starting with a learning rate of 0.1, the data scientist may iteratively modify it to maximize the model’s accuracy.
4. Hyperband overview
Hyperband is a hyperparameter tuning method employing a bandit-based approach to explore the hyperparameter space efficiently.
The Hyperband methodology involves executing a series of “bracketed” trials. The model is also trained in each iteration using various hyperparameter configurations. Model performance is then assessed using a designated metric, such as accuracy or F1 score. The model with the best performance is chosen, and the hyperparameter space is subsequently narrowed to concentrate on the most promising configurations. This iterative process continues until the optimal set of hyperparameters is identified.
5. Random search overview
Random search is a hyperparameter tuning technique that randomly selects hyperparameter combinations from a predefined set, followed by model training using these randomly chosen hyperparameters.
To implement random search, the data scientist or machine learning engineer specifies a set of potential values for each hyperparameter. The algorithm then randomly picks a combination of these values. For instance, if hyperparameters contain the learning rate and all the applicable numbers of hidden layers in a neural network, the random search algorithm might randomly choose a learning rate of 0.1 and two hidden layers.
The model is subsequently trained and evaluated using a specified metric (e.g., accuracy or F1 score). This process is iterated a predefined number of times, and the hyperparameter combination resulting in the best model performance is identified as the optimal set.