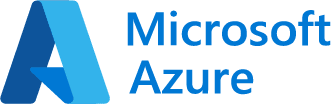
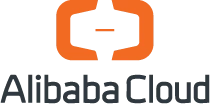
ML Tasks, models, artifacts and datasets
Metrics tracking and visualization
Cost and performance tracking
By focusing on ML tasks, models, artifacts, and datasets, organizations can ensure comprehensive monitoring, logging, and management of ML workflows.
With OptScale, users get full transparency across:
Model tracking involves systematically recording and managing details about machine learning models throughout their lifecycle. OptScale provides users with an in-depth analysis of performance metrics for any API call to PaaS or external SaaS services. Metrics tracking, including CPU, GPU, RAM, inference time, and visualization with multiple tables and graphs, help enhance performance and optimize infrastructure costs.
OptScale Leaderboards gives ML teams full transparency across the ML model metrics, helps compare ML task-run groups against each other based on their performance, and finds the optimal combinations.
OptScale profiles machine learning models and deeply analyzes internal and external metrics for any API call to PaaS or external SaaS services. The platform constantly monitors cost, performance, and output parameters for better ML visibility. Complete transparency helps identify bottlenecks and adjust the algorithm’s parameters to maximize ML/AI training resource utilization and the outcome of experiments.
A full description of OptScale as an MLOps open source platform.
Enhance the ML process in your company with OptScale capabilities, including
Find out how to:
Powered by