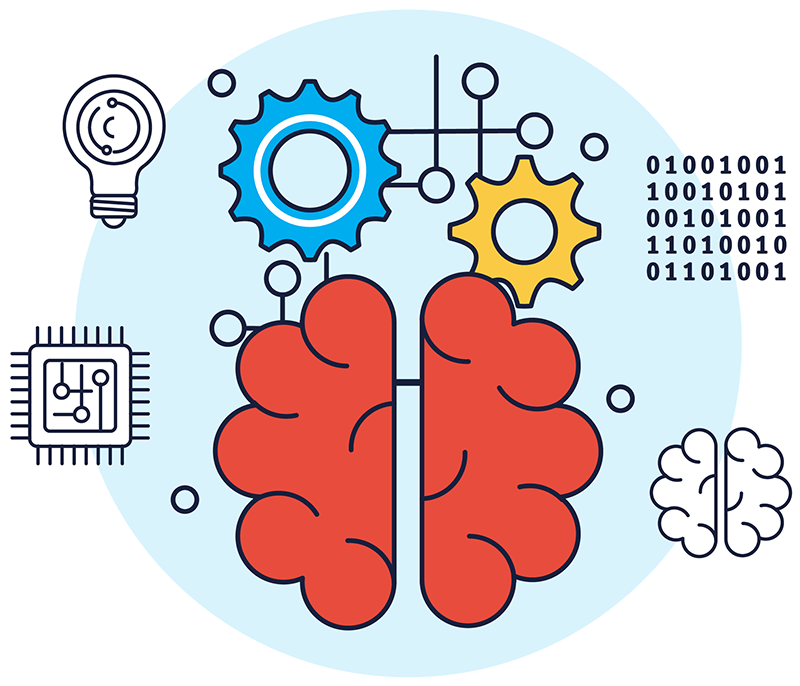
Data is of immense importance in machine learning. A top-notch training dataset is the cornerstone of successful machine-learning endeavors. It significantly impacts the accuracy and efficiency of model training while also playing a pivotal role in ensuring fairness and impartiality in the model’s outcomes. Let’s delve into the best practices and considerations when selecting or preparing a dataset for training machine learning models applicable to structured numerical and unstructured data like images and videos.
Understanding the distribution of the dataset
Examining the dataset’s distribution is crucial, particularly for numerical data. Analyzing the frequency distribution, which illustrates how often each value appears in the dataset, provides valuable insights into the problem at hand and the distribution of classes. ML practitioners usually aim for datasets with a normal distribution to ensure adequate data points for model training.
While a normal distribution is prevalent in natural and psychological phenomena, it’s not a prerequisite for every dataset used in model training. Real-world datasets may not conform to the classic bell curve, and that’s perfectly fine.
Is the data representing reality?
Machine learning models are designed to tackle real-world issues, so it’s essential that the data they’re trained on mirrors reality. While synthetic data can be used when gathering more data is challenging or to balance classes, relying solely on real-world data enhances the model’s robustness during testing and production. Simply inputting random numbers into a machine-learning model won’t magically solve your business problems with 90% accuracy!
Does the data align with the context?
It’s crucial to ensure that the dataset used for model training reflects the conditions the model will encounter in production. For instance, if we’re training a computer vision model for a mobile app that identifies tree leaves from images taken with a mobile camera, more than using images solely captured in a controlled lab environment will be necessary. The training set should include pictures captured in the wild, resembling real-world scenarios the application will face.
Is there data redundancy?
Data redundancy, or duplicative data points, is critical in ML model training. When the dataset contains repeated data points, the model may overfit those points, leading to poor performance during testing (often resulting in underfitting).
Is the data biased?
A biased dataset can never yield an unbiased, trained model. Selecting a balanced dataset that doesn’t favor particular cases is essential. Consider a supervised computer vision model designed to identify gender based on facial features. If the model is trained exclusively on images of individuals from the USA but deployed globally, it will produce unrealistic predictions due to its bias towards a specific ethnicity. The training set should include pictures from diverse ethnicities and age groups to mitigate bias.
Is there an optimal amount of data?
Determining the ideal data for model training takes much work. Deep learning models, in particular, thrive on large datasets to capture complex, nonlinear relationships. However, having too much data can lengthen and inflate the cost of the training process without necessarily improving model accuracy. Too much data can result in overfitting, wherein the model excels on training data but falters on unseen data. Finding the right balance and ensuring enough data from all classes, including edge cases, are vital to train the model effectively.
Optimize your ML/AI costs with Hystax OptScale software: OptScale identifies bottlenecks and provides actionable recommendations to achieve peak performance and cost efficiency → Try it out in OptScale demo