Organizations constantly seek innovative ways to modernize and streamline operations in today’s fast-paced digital landscape. Machine learning (ML) has become a game-changing solution, empowering businesses to automate tasks with unprecedented precision. Unlike traditional rule-based systems, ML thrives in managing complex workflows by continuously learning and adapting, enhancing accuracy and long-term efficiency.
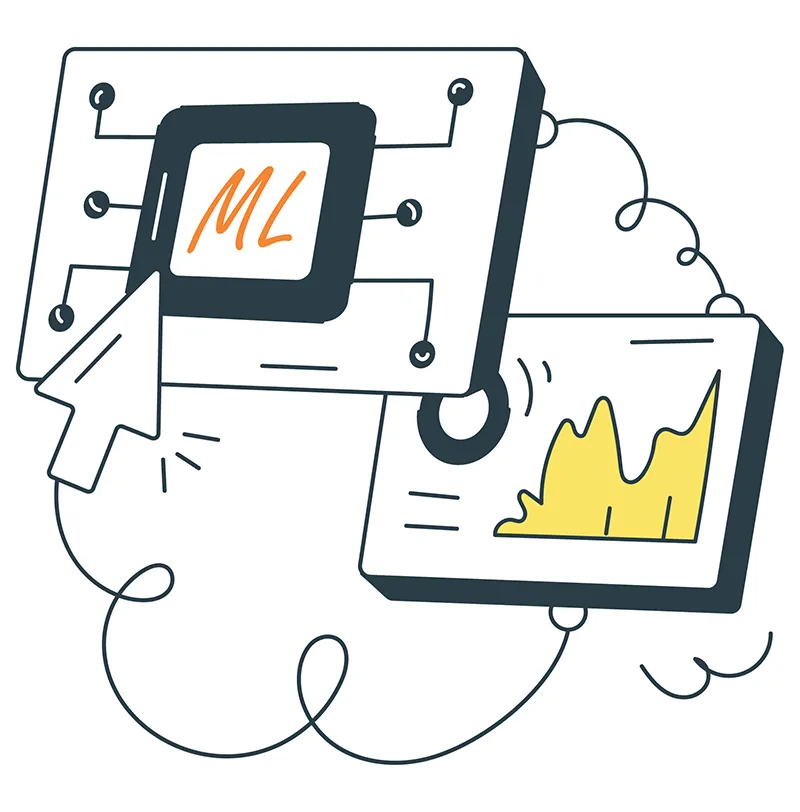
Challenges in scaling machine learning
While machine learning (ML) offers transformative potential, many organizations remain in the pilot phase. Despite developing isolated ML use cases, scaling them across the enterprise proves challenging. A recent survey revealed that only 15% of organizations have successfully scaled automation across multiple business areas, while just 36% have moved ML algorithms beyond the pilot stage.
This slow progress often stems from incomplete documentation of institutional process knowledge, making it challenging to capture decision-making with simple rule sets. Additionally, resources on scaling ML are usually too abstract or overly technical, leaving business leaders without actionable guidance to drive adoption effectively.
Unlocking value through machine learning
Adopting ML at scale presents substantial opportunities for businesses. Leading organizations have reported process efficiency increases exceeding 30% and revenue growth of 5–10%. For example, a healthcare company implemented a predictive model to classify claims by risk category, achieving a 30% boost in automatic claims processing and a 25% reduction in manual effort.
By embedding ML into their operations, companies can develop scalable, resilient systems that deliver sustained value. This strategy will help them maintain a competitive advantage in a dynamic market.
Key takeaways for leveraging machine learning
To maximize the benefits of machine learning (ML) and streamline processes, organizations should focus on these strategies:
Preserve institutional knowledge
Systematically document key process insights to ensure you effectively integrate them into ML models and workflows.
Focus on practical implementation
Opt for actionable, user-friendly resources to empower all team members, including non-technical staff, to scale ML initiatives successfully.
Advance beyond pilot projects
Transitioning from isolated ML use cases to scaling automation across diverse business functions drives enterprise-wide impact.
Measure and refine outcomes
Regularly track ML’s impact on efficiency and revenue to optimize performance and demonstrate ROI.
Embrace complexity
Leverage ML’s capability to manage intricate processes and nuanced decision-making, areas where traditional methods fall short.
Create scalable, resilient systems
Build adaptive ML-driven systems that evolve with changing demands, ensuring long-term value and competitive advantage.
How to drive impact with machine learning: A four-step strategy
Machine learning (ML) is revolutionizing industries, but its rapid advancement can overwhelm leaders. To navigate this evolving landscape, successful organizations integrate ML seamlessly into their operations using a streamlined, four-step approach.
Step 1: Build economies of scale and expertise
A common pitfall when operationalizing ML is focusing on isolated steps managed by individual teams. This fragmented strategy limits scalability and strains resources. Instead, organizations can maximize ML’s potential by fostering collaboration and adopting a comprehensive perspective on automation.
- Eliminate silos: Encourage cross-functional collaboration to ensure ML initiatives move beyond pilot projects. This approach tackles critical challenges like model integration and data governance.
- Design end-to-end automation: To drive efficiency, shift the focus from isolated tasks to automating workflows. Identify shared elements such as data inputs, review protocols, processing steps, and documentation.
- Leverage similarities across use cases: Identify patterns across use cases, like document processing or anomaly detection, to implement ML at scale and capitalize on synergies.
Organizations can achieve economies of scale by prioritizing collaboration and taking a holistic approach, making ML implementation more impactful and efficient.
This strategy streamlines processes and positions businesses to remain competitive in an ever-evolving marketplace.
Step 2: Assessing capability needs and development strategies
The second step in implementing machine learning (ML) involves identifying the specific capabilities required based on the archetype use cases identified earlier. For example:
- Companies looking to strengthen controls might focus on anomaly detection capabilities.
- Organizations facing challenges with digital channel migration may prioritize natural language processing and text extraction technologies.
ML model development approaches
To build the necessary ML models, organizations can choose from three primary strategies:
- Internal development
Developing fully customized ML models internally offers tailored solutions to meet unique requirements. However, this approach requires significant time, expertise, and resources. - Platform-centric solutions
Leveraging low- or no-code platforms streamlines the ML development process, allowing faster implementation without extensive coding expertise. This option is ideal for businesses seeking efficiency and scalability. - Pre-built point solutions
Purchasing ready-made ML solutions designed for specific use cases allows quick implementation. While convenient, this approach may involve trade-offs in flexibility and customization.
Key considerations
For basic transactional processes, such as those in banking operations, platform-based solutions often provide the best balance of speed, cost, and capability. By thoroughly analyzing these factors, businesses can make informed decisions aligned with their strategic goals, ensuring the successful adoption of ML technologies.
Step 3: Training machine learning models in real-world settings
A critical phase in operationalizing machine learning (ML) is providing models with practical, real-world training to enhance their knowledge and accuracy. This step focuses on enabling models to analyze quality data and adapt effectively, but it also involves overcoming specific challenges.
Key considerations for model training
Ensuring high-quality data is foundational for successful ML training. Challenges include:
1. Managing data from multiple legacy systems.
2. Cleaning and maintaining datasets consistently across the organization.
2. Sequential training environments
ML training typically spans three distinct environments:
1. Developer environment: Systems are created and easily modified.
2. Test environment: Users test functionalities with limited system modifications (user-acceptance testing or UAT).
3. Production environment: Systems operate at scale, providing real-world data for optimal learning and adaptation.
4. Regulatory and privacy constraints: The availability of real-world data across contexts may be restricted by privacy concerns in highly regulated businesses like banking or healthcare. Striking a balance between data access and compliance is essential for practical training.
3. Optimizing training in production environments
Training ML models in production environments is often the most effective approach, as it exposes them to real-world conditions. However, organizations must incorporate safeguards to address regulatory constraints and privacy concerns.
1. Human-in-the-loop approach: To mitigate risks, leading organizations implement human oversight during the training process. This approach involves setting decision thresholds and reviewing model outputs before granting full autonomy.
2. Gradual autonomy: Models gain independence only after surpassing predefined accuracy thresholds, ensuring reliable performance.
Practical example: A healthcare success story
One healthcare company adopted this methodology and achieved remarkable results. Over three months, it enhanced the accuracy of its ML model, increasing straight-through processing rates from under 40% to over 80%. By combining human oversight with machine learning capabilities, the organization significantly improved efficiency while maintaining control over decision-making.
By focusing on real-world training, managing data quality, and addressing regulatory requirements, organizations can empower ML models to deliver consistent, high-impact results in real-world applications.
Step 4: Optimizing machine learning projects for deployment and scalability
Successfully deploying and scaling machine learning (ML) projects requires a standardized approach that fosters consistency and maximizes impact. Organizations can streamline their ML initiatives and unlock their full potential by focusing on key principles.
Key strategies for standardizing ML projects
1. Cultivate a learning culture
Treat ML projects as opportunities for experimentation and learning, much like scientific research. Even unsuccessful experiments provide valuable insights that can refine future efforts and improve long-term ML capabilities.
2. Adopt MLOps best practices
Inspired by DevOps, MLOps (Machine Learning Operations) integrates software development and IT operations principles into the ML lifecycle. This integration includes:
- Automating repetitive tasks in data engineering and data science workflows.
- Enhancing model stability and reproducibility.
- Ensuring consistency across development, testing, and deployment phases.
3. Automate and standardize workflows
Automation is essential for reducing human error, enhancing collaboration, and accelerating ML project timelines. Standardizing workflows facilitates seamless knowledge sharing and enables different teams to work efficiently toward common goals.
4. Enhance deployment and scalability
Standardized processes and automated tools are vital for efficient ML model deployment and scaling. They ensure smooth integration into existing systems and reliable operation at scale, enabling businesses to handle increased demands effectively.
Achieving impactful ML deployments
By embracing standardization and automation, organizations can:
- Deploy ML models consistently across various applications.
- Scale ML systems seamlessly to meet growing business needs.
- Realize reliable, high-impact solutions that deliver sustained value.
Standardized processes improve efficiency and create a foundation for scalability, ensuring ML models are well-positioned to drive meaningful outcomes across the organization.
Summary
Machine learning can optimize business processes to drive efficiency and spur innovation. By automating complex tasks and streamlining workflows, ML helps reduce costs and enhances operational performance across diverse industries. Real-world examples and actionable strategies illustrate how embracing ML transforms traditional operations into dynamic, growth-oriented systems.
✅ ML Experiment Tracking: Definition, Benefits, and Best Practices → https://optscale.ai/experiment-tracking-in-machine-learning/