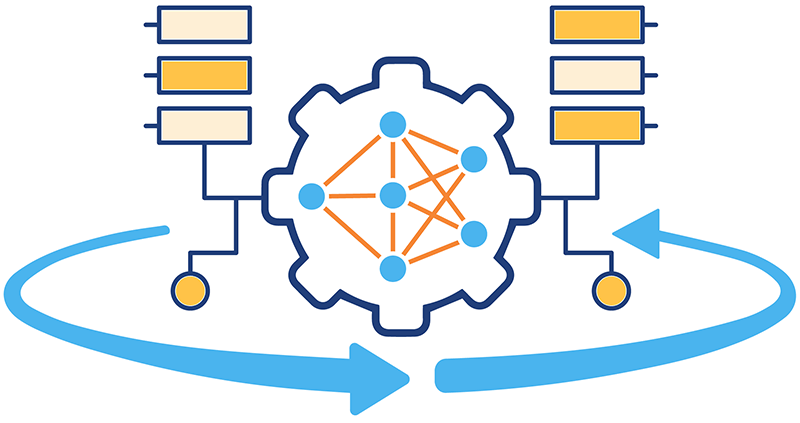
Picture a world where artificial intelligence (AI) and Machine Learning (ML) are woven into the fabric of our daily lives. As this technological wave continues to surge, adopting MLOps best practices has turned from a good idea into an absolute necessity. These practices are like the guiding stars that ensure AI and ML systems not only grow efficiently but also remain trustworthy and reliable.
Now, imagine the scene: discussions buzzing about fresh regulations aimed at taming the realm of AI, just like the European AI strategy. These regulations are like the rules of the road for AI applications in the real world. And guess what? They’re shining a bright spotlight on something called “model governance.” Suddenly, companies, big and small, are realizing that it’s time to get their model governance game on point.
So, what exactly is model governance? Well, it’s like the responsible guardian of the AI realm. It ensures that AI systems behave themselves, following the rules and being accountable for their actions. And when model governance teams up with MLOps processes, which we’ll call MLOps governance, magic happens. Think of MLOps governance as the ultimate tag team that brings many benefits to the ring.
From the tech side, MLOps governance acts as a safety net. It minimizes risks and maximizes the quality of ML systems strutting their stuff in the real world. On the legal front, it’s the superhero cape that ensures these systems play by the rules set by the regulators. Plus, it’s like a badge of honor, showing everyone that compliance is not just a word – it’s a way of operating.
Now, let’s peek at what this article has in store. First up, we’re going to unravel the mysteries of model governance and its partner in crime, MLOps governance. We’ll chat about why MLOps governance has taken the spotlight. And to keep things practical, we’ll dive into how you can set up your governance framework. This framework isn’t just about rules; it’s also about the nitty-gritty processes that make MLOps tick. So, buckle up as we journey through AI responsibility and reliability.
Exploring the concept of model governance
Understanding model governance
Model governance in AI/ML is about having processes to track how our models are used. It’s like following a set of rules.
Connection with MLOps
Model governance and MLOps (managing Machine Learning operations) go hand in hand. How much governance we need depends on how many models we use and the rules in our field.
Variation in integration
The way we blend ML model governance with MLOps can be different based on factors like the number of models in action and the regulations in our business domain.
More models, more governance
If we’re using many models, ML model governance becomes a crucial part of how we handle MLOps.
Core of ML system
Think of it this way – as we use MLOps governance more, it becomes a central part of how our entire Machine Learning setup works. It’s like the heart of the system.
MLOps governance as the ever-reliable co-pilot on your Machine Learning expedition. It’s not just a bunch of rules; it’s your sidekick ensuring that every step of your model’s journey, from creation to deployment, is as smooth as silk. Let’s break down this dynamic partnership into its key phases:
Phase 1: Creative exploration
In the early stages of model development and experimentation, MLOps governance shines by maintaining a digital breadcrumb trail. It’s like leaving a trail of glow-in-the-dark paint so you can always retrace your steps. Plus, it’s got a knack for resource sharing, making collaboration a breeze within your team. Think of it as the tech-savvy Sherlock Holmes of your project.
Phase 2: The Grand debut
When your model is ready to hit the real world, MLOps governance steps up like a vigilant butler. It oversees the performance, making sure your model behaves its best. Security? Covered. Documentation? Present and clear. It’s as if your model has its entourage of experts handling the backstage details while your creation takes the spotlight.
The twin pillars: Data and Model Management
Imagine MLOps governance as a grand palace with two majestic towers: Data Management and Model Management. The first tower guards your data, ensuring it’s handled with care and respect for your organization’s guidelines. The second tower takes charge of the models, their code, and the pipelines, orchestrating a harmonious symphony of efficiency.
So, next time you dive into MLOps governance, envision it as your trusty co-pilot and a grand palace all rolled into one. It’s the art of keeping things shipshape in Machine Learning, letting you focus on the magic of creation while it handles the mechanics.
Why does MLOps governance matter?
MLOps model governance brings an elevated level of control and transparency to the functioning of ML models and pipelines in real-world scenarios tailored to different stakeholders’ needs. This structured ability to trace activities brings forth a host of advantages:
Optimizing model performance
By swiftly pinpointing and addressing bugs and glitches, it ensures that models perform at their best once they are deployed.
Ensuring fairness
Through explainability features, it aids in the ongoing assurance of fairness in models by detecting and mitigating biases.
Maintaining comprehensive audits
It seamlessly documents the journey of models, providing a complete audit trail that aids in analysis and understanding.
Spotting and tackling potential risks
It allows for the swift identification and resolution of possible risks tied to ML, such as using sensitive data or inadvertently excluding certain user groups.
This reliable capability to carry out these functions can genuinely impact the triumph of any ML endeavor, particularly those of extended duration. The influence goes beyond adhering to regulations and following superior engineering techniques; it extends to burnishing reputation and delivering enhanced model performance for the end users.
Understanding a model governance framework
A model governance framework encompasses all the systems and processes in place to fulfill the model governance needs for every operational Machine Learning model. During the initial phases of adopting Machine Learning, this governance might be carried out manually, lacking streamlined tools and methods. Although this manual approach can be fitting initially, it falls short of establishing a solid foundation for effective governance as the team and its procedures mature. It also hinders the scalability of Machine Learning across numerous models.
Crafting a model governance framework for Machine Learning isn’t a straightforward endeavor. Given the relatively new nature of this field and the ever-evolving regulatory landscape, defining such a framework poses challenges and requires a keen eye on changing requirements.
Laying the foundation for a model governance framework
The process of setting up a model governance framework can be broken down into distinct phases:
Assessing regulatory compliance
Different types of Machine Learning applications often come with their own set of rules and regulations. When setting up a new production pipeline for machine learning models or when giving an existing one a once-over, there are some critical steps to keep in mind:
- Understand the ML context: First, get to know the specific category of Machine Learning you’re dealing with. Each area might have unique regulatory requirements.
- Identify the guardians: Figure out who will be responsible for keeping an eye on the governance processes. These are the folks who’ll make sure everything runs smoothly.
- Define the rules: Get your policies in order. This means thinking about things like personal identifiable information (PPI), special regulations for your field, and any regional rules that apply.
- Blend with MLOps: Integrate these rules into your MLOps platform. This ensures that the regulations are woven into the very fabric of your model’s life cycle.
- Engage and educate: Bring everyone on board. Make sure all the stakeholders know what’s going on and why it matters. Education is vital to successful governance.
- Keep a watchful eye: Like a vigilant guardian, keep tabs on how things are going. Regularly monitor and refine your processes to ensure your model stays in tune with the rules as they evolve.
With these steps, your journey towards effective model governance becomes a well-guided adventure, where rules and regulations are not hurdles but rather the wind in your sails.
Enabling model governance through MLOps
So, MLOps governance isn’t just a rulebook; it’s a symphony of tools and practices, ensuring that data and models pirouette smoothly on the stage of success.
💫 What are the driving factors for MLOps? Explore the motivation behind MLOps, the overlapping issues between MLOps and DevOps, and many other essential things → https://optscale.ai/why-mlops-matters-bridging-the-gap-between-machine-learning-and-operations/
✔️ Do you want your cloud and ML/AI operations to be under control and your expenses to meet your expectations? Assess the capabilities and potential of an open source platform OptScale → https://optscale.ai/introducing-optscale-public-release-an-open-source-powerhouse-for-finops-and-mlops/