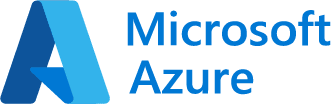
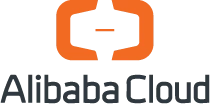
The RI/SP usage dashboard allows OptScale users to forecast guaranteed usage, utilize recommendations for better RI/SP utilization, and save a double-digit percentage of monthly cloud spend.
By integrating with an ML/AI model training process, OptScale highlights bottlenecks and offers clear recommendations to reach ML/AI performance optimization.
The recommendations include utilizing Reserved/Spot instances and Savings Plans, which help minimize cloud costs for ML/AI experiments and development.
Strategically combining Savings Plans, Reserved Instances, and Spot Instances enables organizations to strike a balance between cost efficiency and flexibility, maximizing the value of machine learning processes.
By integrated profiling, OptScale highlights bottlenecks of every experiment run and offers clear optimization recommendations to reach performance enhancement. The recommendations include utilizing Reserved/Spot Instances and Savings Plans, rightsizing and instance family migration, and detecting CPU/IO and IOPS inconsistencies caused by data transformations or model code inefficiencies.
Unused and overlooked resources are contributed to a company cloud bill, and users don’t even expect that they’re paying for them.
OptScale allows ML specialists to identify and clean up orphaned snapshots to keep cloud costs under control.
OptScale’s Power Schedules feature allows the scheduled shutdown of instances; users can automatically start and stop VMs to avoid the risk of human error and the burden of manual management.
With a single solution that works across multiple cloud platforms, customers have a consistent and streamlined experience, no matter where their resources are hosted.
The Duplicate Object Finder for AWS S3 delivers significant cloud cost reduction by illuminating the duplicated objects.
The labyrinth of AWS S3 often houses duplicate objects, a silent menace that, over time, bloats cloud expenditures. OptScale scans and fishes out duplicates from a great number of S3 objects. Dispelling the constraints of singular account connectivity, OptScale’s Duplicate S3 Object Finder empowers users to link unlimited AWS cloud accounts.
Choosing the optimal instance type and family involves selecting VMs from a cloud provider’s offerings that best meet the performance and cost requirements of your ML workloads.
OptScale enables balancing performance needs with cost considerations by selecting instances that provide the required resources at the lowest cost.
By continuously monitoring, analyzing, and adjusting VM configurations based on workload requirements, OptScale enhances the performance of ML workflows while minimizing costs.
With OptScale, users Improve visibility and control over Databricks expenses and get details on which experiments the costs are distributed
Supporting Databricks by the OptScale platform allows ML specialists to identify how the Databricks costs are distributed across ML experiments and tasks. The connected Databricks data source is managed in the same way as other data sources.
OptScale captures metadata from Databricks resources, such as name, tags, and region, enabling effective cost allocation.
With OptScale, users get the complete picture of S3 and Redshift API calls, usage, and cost for their ML model training or data engineering experiments. The platform provides users with metrics tracking and visualization, as well as performance and cost optimization recommendations.
A full description of OptScale as an MLOps open source platform.
Enhance the ML process in your company with OptScale capabilities, including
Find out how to:
Powered by