Cloud cost optimization is a topic keeping many cloud users up at night. Who wouldn’t want to take advice and recommendations from experts then? The same is true for today’s cloud users. Companies investing and utilizing the cloud should be turning to machine generated recommendations for their virtual machine (VM) instances. Those who do are both cutting cloud costs and optimizing the way they use the cloud across their companies and teams.
Rightsizing is often a huge challenge that companies face. Having access to rightsizing recommendations to consider can really help businesses leaders and FinOps teams to solve and improve their companies’ current processes and cloud usage.
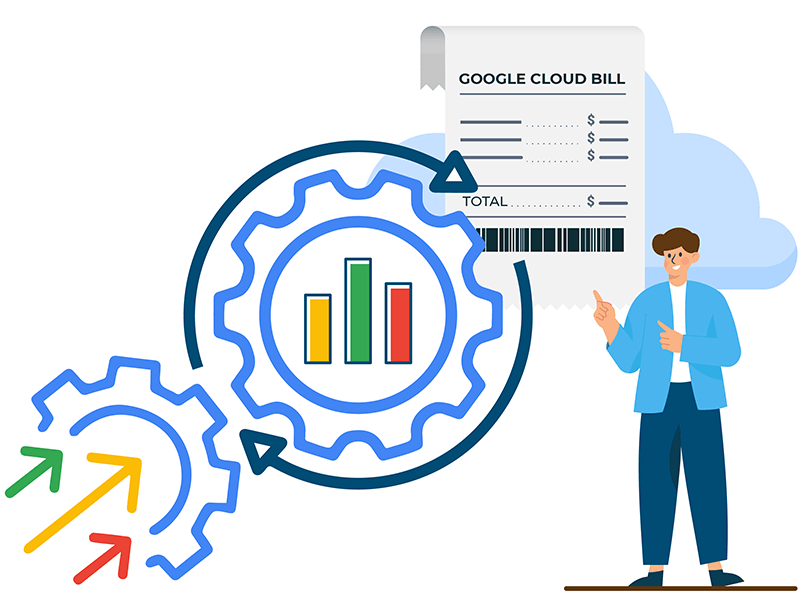
With many companies enlisting a multi-cloud strategy today, Google Cloud Platform (GCP) is used by many of them. However, optimization is easier said than done on the platform. Google Cloud Platform cost optimization can be achieved with ongoing monitoring and accurate forecasting, but it can be made all that much easier when a Compute Engine provides machine type recommendations. These bits of advice can help GCP users optimize how they use and allocate resources of their VM instances.
This advice is generated automatically. It is based on system metrics which collect Cloud Monitoring service data over the previous 8 days. Companies can then apply these recommendations to resize their instance types to use the resources more effectively. Rightsizing recommendations can therefore be a gamechanger.
What are rightsizing recommendations really?
Rightsizing recommendations features simply help cloud professionals pinpoint cost-saving opportunities. They showcase the ability to downsize or remove instances.
Rightsizing recommendations deeply analyze your resources and usage to spotlight opportunities to reduce cloud costs. Using this feature, companies can see all of their underutilized instances across their various member accounts but within one single view. This capability allows them to be served up recommendations that are easy to understand on how they can save, as well as how much savings they can expect in some cases. Rightsizing recommendations open their eyes and provide cloud cost transparency on items they might be missing so that they can take action and make adjustments quickly.
The biggest reasons to consider rightsizing recommendations
Many cloud cost management and cloud cost optimization opportunities come with considering rightsizing recs.
What it comes down to is offering a surefire way to receive estimated savings percentages, estimated savings per month, and pure opportunities for optimization. Of course, it all pertains to your usage and resources. These recommendations help GCP users, for example, make adjustments more seamlessly within the platform and prevent cloud bill surprises.
What to know in terms of price and limitations
Users can receive machine type recommendations for free. However, when they act and apply a recommendation to resize their instance type, they are then billed for the machine type that is chosen.
It’s also worth noting that there are some use cases where machine type recommendations aren’t available. These limitations apply when considering VM instances designed with App Engine flexible environments, Dataflow or Google Kubernetes Engine. It also is limited when it comes to VM instances with ephemeral disks, GPUs or TPUs.
When rightsizing recommendations work well
The algorithm for cloud optimization recommendations regarding rightsizing works well with workloads that follow weekly cadences. It also performs well on those which grow or shrink over weeks of time, workloads that underutilize resources consistently and in many other scenarios. In all of these events, having eight days worth of data is enough to make predictions on how to best alter the size of the machine to improve your resource use.
For Google Cloud users looking for GCP cost optimization through rightsizing, here is a helpful article on available machine types for VM instances.
How do machine type recommendations work?
The recommendations are generated in the following way. A Compute Engine tracks the CPU and memory usage of running VMs. It then makes recommendations using data from the last eight days.
The Compute Engine then splurts out specific recommendations according to that data. These can range from recommendations for a machine type with fewer virtual CPUs should an instance have low CPU utilization the majority of the time. If the majority of the time it’s running, high CPU usage is seen, suggestions are likely to be made to consider more virtual CPUs.
Additional recommendations can be made based on memory usage as well to recommend less or more based on these eight data days.
Becoming familiar with Recommender on GCP
GCP Recommender offers users a better way to think about and act when it comes to cloud cost management. It is based on some key pillars, which range from more obvious to proactive considerations.
These pillars include:
- Cost
- Security
- Performance
- Reliability
- Manageability
- Sustainability
Recommenders on Google Cloud offer usage recommendations for GC resources. They are usually specific and consider a single product and resource type. Each single product can come with various recommenders as well.
These tips can include everything from recommendations to remove unused SQL instances to resizing advice on overprovisioned instances to protecting your account and removing access permissions. Users can also receive notifications for Kubernetes deprecations or recommendations to reclaim or remove projects which are doing unused.
Putting recommendations into action
GCP users can apply, disregard or restore recommendations from the Recommendation Hub. However, it’s important to note and Google says this disclaimer on its own site that “before applying recommendations in the Google Cloud console or using the API, ensure that impacts are assessed by a reviewer.”
While the data is strong and the algorithms are reliable, it’s always great to have a reviewer on your end who has the knowledge to delve into the impact laid out in recommendations, plus understand how they play into your infrastructure and company.
For recommendations that your team is behind, there is a simple Apply button that appears in the recommendation details panel. You’ll also have the option to dismiss recommendations. When you dismiss them, it prevents these tips from showing up in your Recommendation Hub dashboard, plus the list views for your project.
Some extra help with Hystax OptScale
In addition to all of the helpful tools that GCP’s recommendations provide, rarely are cloud users using GCP and looking for cloud cost optimization ideas on that platform alone. When looking for chances to optimize your overall cloud usage, you want the whole picture and transparency in areas where the most significant spending is happening. This spend is usually attributed to VMs and their persistent disks. Having access to this information and more as you work with the cloud across teams, users, different privileges, and more can be harder to uncover than it needs to be at times.
OptScale can be a helpful solution for bringing full transparency into your GCP spending and those on other clouds. It provides hundreds of cost-saving and optimization recommendations that its users can significantly benefit from.
The recommendations are fruitful and many, from VM rightsizing and an object storage duplicate finder to abandoned bucket optimization. Moreover, users can really gain insights into how to control their cloud usage or optimize it if they have a multi-cloud infrastructure in place.
The ability to cut cloud costs but still utilize it to its full potential is something every business leader is after. Contact us to better understand your cloud consumption, optimize cloud costs, and more. We’d be happy to provide tips on how to go about looking at GCP rightsizing recommendations, our overall optimization recommendations, and much more to offer you support on your cloud strategy and the ongoing tweaks being made.
Click here to read more about how Hystax offers the first-ever open source FinOps and multi-cloud cost management solution.
💡 Discover how recommendation cards in OptScale help enhance cloud resource management and reduce overspending using Google Cloud → https://optscale.ai/how-to-use-recommendation-cards-in-optscale/